About Sterblue
Sterblue builds software that manages infrastructure inspection. Instead of dispatching crews of people to climb up power lines or wind turbines, Sterblue’s software analyzes imagery from drones, helicopters, satellites, and smartphones to find defects. This allows utility companies to find defects in their infrastructure much more cheaply, quickly, and safely. Sterblue provides functionality for planning drone flights, ingesting and managing visual data, and extracting insights from this data. Infrastructure inspections can be managed within a single platform that provides a streamlined workflow suitable for enterprise customers.
Sterblue serves a collection of international enterprise customers that manage infrastructure including power lines, wind turbines, cooling towers, and more. The company graduated from the prestigious YCombinator startup accelerator, employs 26 people, and is based in Nantes, France.
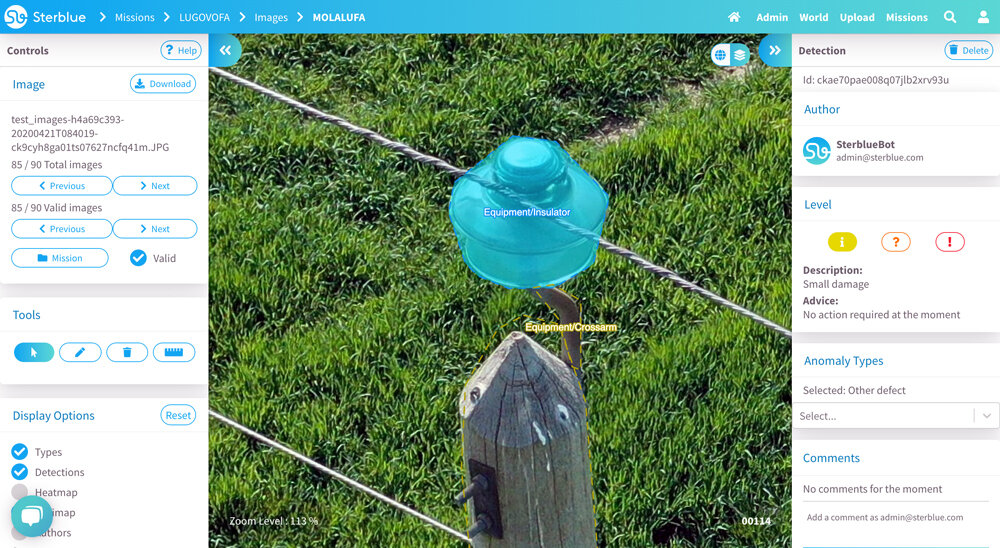
Goals
Sterblue utilizes a combination of artificial intelligence tools and business domain experts to find anomalies from images and output reports with actionable insights for their users. They manage a variety of datasets across different task types and customer domains, and must be able to optimize their artificial intelligence tools to provide high quality insights to their customers at a reasonable cost.
To provide a valuable service for their customers, Sterblue optimizes the following attributes of their defect detection system:
- High detection accuracy: Producing accurate anomaly detection that is as good or better than customers’ existing processes.
- High data throughput: Customers produce large datasets of images, and Sterblue must be able to quickly sift through these images to produce insights.
- Reduced human involvement: To provide a service that is more efficient than current processes, Sterblue must be able to leverage automation to reduce the amount of manual inspection needed from human experts.
Using Aquarium
Sterblue has partnered with Aquarium to improve the quality of the machine learning models they use for anomaly detection. Aquarium's machine learning data management platform makes it easy to inspect label quality and model performance, then take actions to improve model performance by improving the data the models are trained on. By providing detailed analysis of model disagreements with labels, teams using Aquarium are able to pinpoint issues much more quickly without needing to manually inspect millions of datapoints.
Sterblue’s machine learning team was able to use Aquarium to quickly find numerous label quality issues, build workflows to fix these label quality issues, and then retrain a significantly more accurate model.
- Sterblue is able to view their labeled datasets at a glance, plot the geographic distribution of where their data was collected, and query for data of interest within Aquarium.
- By analyzing areas where their model disagreed most with their labels, the Sterblue team was able to quickly pinpoint areas where their labels were incorrect.
- Aquarium’s issue tracking workflows integrate into Sterblue’s in-house labeling system, allowing the team to quickly fix label quality issues and retrain their model on the cleaned dataset.
- Aquarium connects to Sterblue’s model training pipeline, allowing the machine learning team to analyze and compare models as soon as they are trained, then seamlessly share the results with stakeholders.
Thanks to Aquarium, Sterblue has one central platform on which we can curate our data and find issues in the datasets.
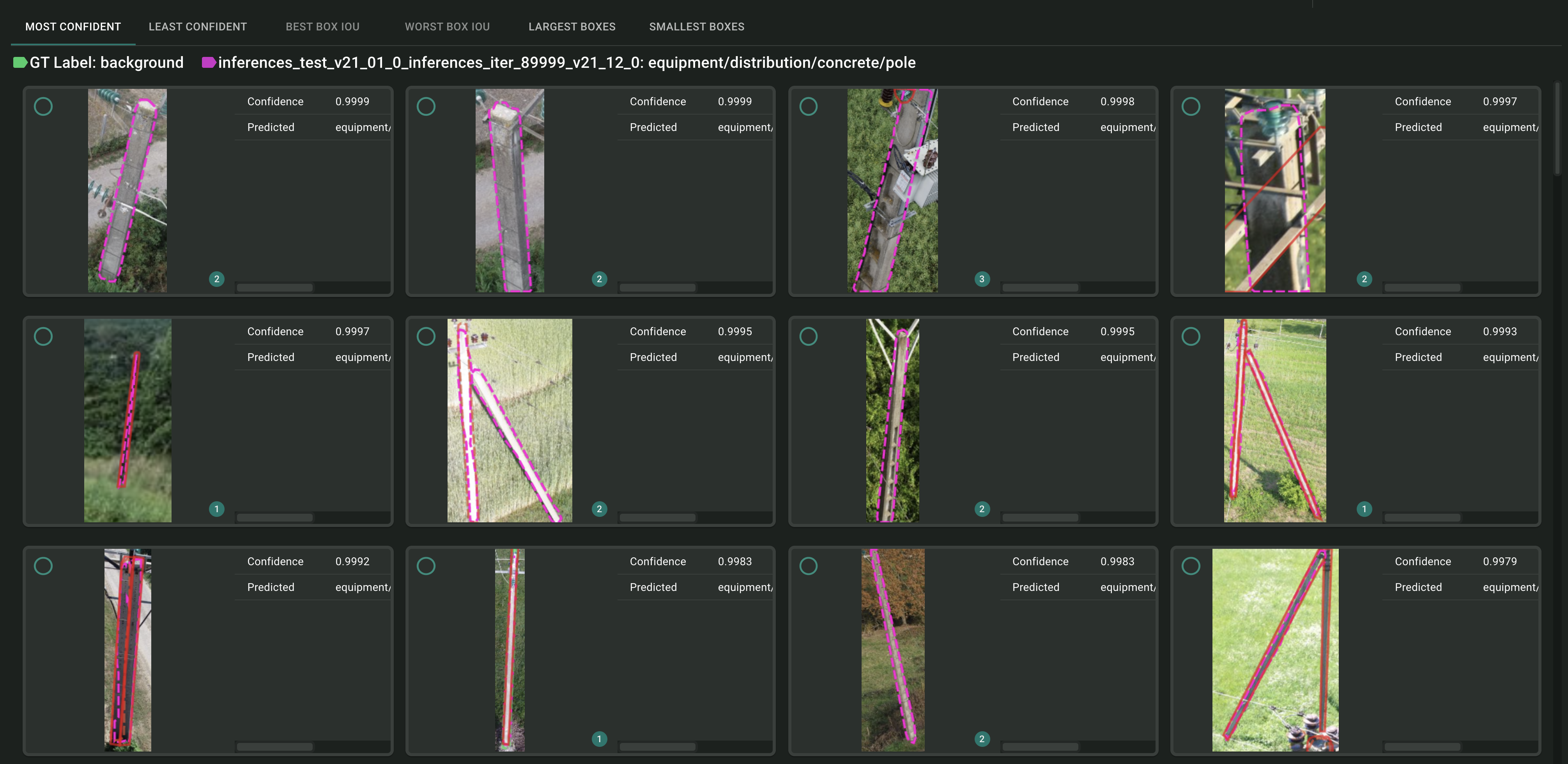
Results
By using Aquarium to accelerate their model improvement, Sterblue was able to deploy a new defect detection model that was 13% more accurate than the previous version. The Sterblue ML team was able to save both engineering and labeling time by using Aquarium's streamlined interface to detect and resolve issues in label quality, yielding large improvements to model performance much more quickly.
These improvements allowed Sterblue to pass on the following benefits for their customers:
- Deliver higher accuracy defect detection.
- Efficiently scale defect detection to several hundred miles worth of power lines.
- Reduce manual inspection time from experts by 50%.