About Winnow
Winnow develops AI technology to help commercial kitchens reduce their food waste. Winnow helps their customers gain insight into what food is being thrown away, allowing them to modify their food production to reduce future food waste. Their customers include household names like IKEA, Hilton, and Compass Group.
Winnow’s AI product, Winnow Vision, uses computer vision to automatically identify what food is thrown away in their customer’s kitchens and in what quantity. By aggregating this data, Winnow can provide actionable analytics to kitchen managers that allow them to cut their food costs by 2-8% per year and reduce their CO2 emissions in the process.
Goals
In the initial days of a customer deployment, Winnow Vision relied on manual categorizations of what type of food was being thrown away. Once enough data had been accumulated, they trained specific deep learning models for each customer.
However, customers had a “waiting period” until enough data had been collected from their kitchen to get the benefits of AI (ie. less human input to record food waste and more accurate analytics). Winnow wanted to develop a new AI model that could be used from day one for any new customer.
Using Aquarium
To create a model that could be used from day one, the Winnow team would have to recategorize hundreds of thousands of images into a uniform taxonomy that could be used for model training. With their previous workflow, this would have taken years of time, making this project infeasible to accomplish.
Aquarium allowed the Winnow team to massively speed up their data curation workflows. Instead of slowly browsing through images one-by-one in a spreadsheet, the Winnow team used Aquarium’s neural network embedding technology to quickly categorize large clusters of similar imagery. They then exported data from Aquarium to an external workforce to do a fine-grained QA pass on their exported imagery, making sure that everything in a category belonged there.
With Aquarium, creating a massive dataset with a unified taxonomy could be accomplished in weeks rather than years.
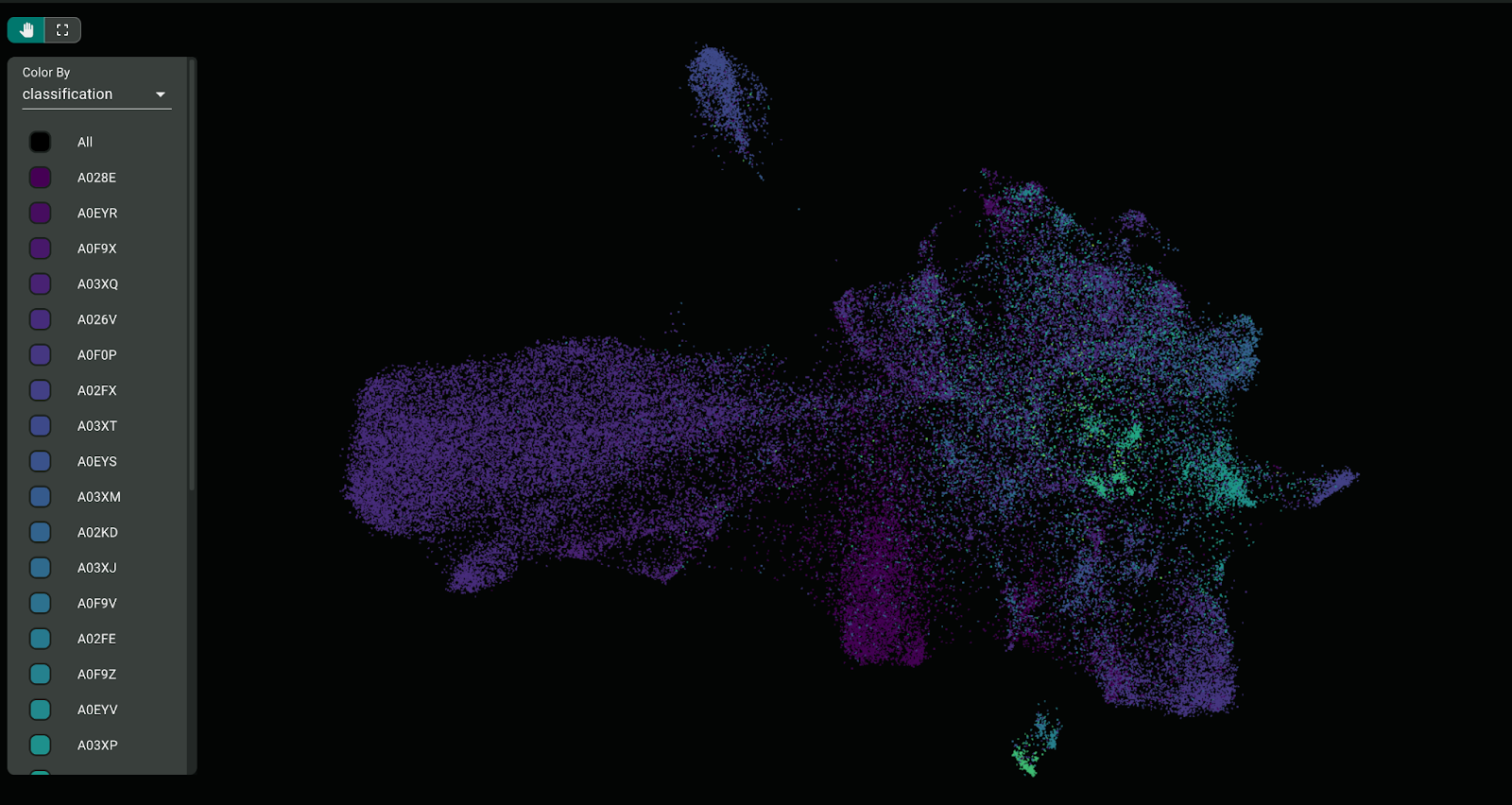
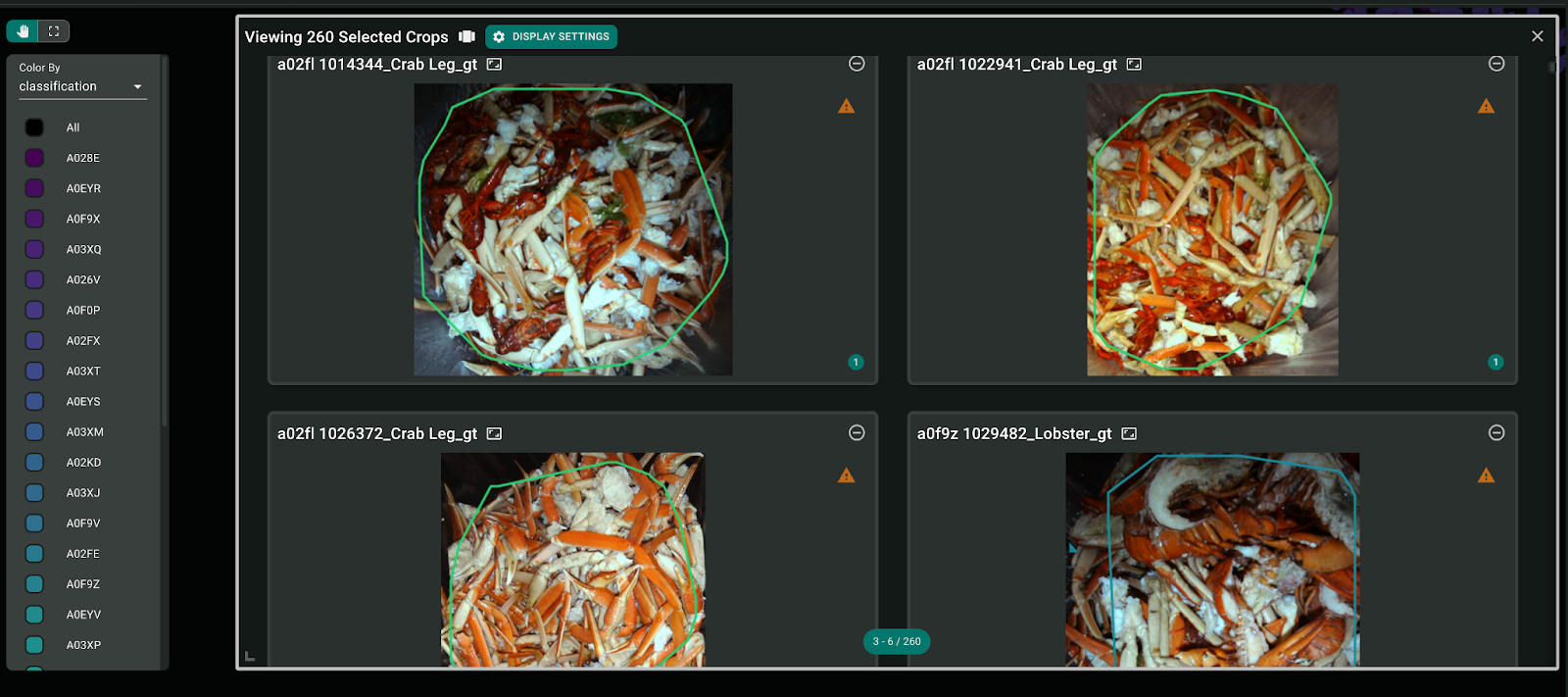
Impact
Over the course of two months, the Winnow data analytics team was able to sort through more than 400,000 images to create a unified dataset consisting of more than 500 classes. They were able to train a single AI model on this unified dataset (as opposed to one model per customer) that was very accurate on their test set metrics.
When testing this model on live customer data, the Winnow team found that the new model was actually more accurate than the human-created annotations in their evaluation datasets. Even better, they saw that this model was significantly more accurate in categorizing food for each customer than most of their customer-specific models.
After a detailed investigation that convinced the Winnow team that this model was a clear improvement over their previous models, they pushed it into production. As a result of this deployment, the Winnow team was able to:
- Reduce the time to value to new customers by providing accurate and automated food categorizations from day one.
- Cut the number of unique models running in production from 12 to 1, allowing them to spend engineering bandwidth on higher-value projects instead of routine model maintenance.
- Improve the accuracy of their system by 15% over their previous customer-specific models.
- Create a much more attractive product that allowed them to acquire more new customers and therefore save more food waste!
“Aquarium helped our machine learning team move much faster, enabling us to deliver on product initiatives that we previously thought weren’t possible. It’s also made a significant impact on our company - we can now onboard new customers far more quickly and win more business than we could before.”
Marc Zornes, Founder and CEO